一文搞定生信分析强助攻-药敏分析(pRRophetic和oncoPredict包)
if (!requireNamespace("BiocManager", quietly = TRUE))
"BiocManager") install.packages(
"limma", "car", "ridge", "preprocessCore", "genefilter", "sva")) BiocManager::install(c(
"BiocManager" 包来安装多个其他包,包括 "limma"、"car"、"ridge"、"preprocessCore"、"genefilter" 和 "sva"。 使用
"ggplot2") install.packages(
"ggpubr") install.packages(
引用包
library(limma)
library(ggpubr)
library(pRRophetic)

library(ggplot2)
#加载所需的R包,包括 "limma"、"ggpubr"、"pRRophetic"、"ggplot2" 等
set.seed(12345)
pFilter=0.001 #pvalue的过滤条件设置显著性过滤条件,用于筛选差异显著的结果
gene="VCAN" #目标基因
expFile="symbol.txt" #表达数据文件
setwd("") #设置工作目录
#获取药物列表
data(cgp2016ExprRma)
#载入已经提供的 "cgp2016ExprRma" 数据集
data(PANCANCER_IC_Tue_Aug_9_15_28_57_2016)
allDrugs=unique(drugData2016$Drug.name)
#载入已经提供的 "PANCANCER_IC_Tue_Aug_9_15_28_57_2016" 数据集。
#读取表达输入文件,并对数据进行处理
rt=read.table(expFile, header=T, sep="t", check.names=F)
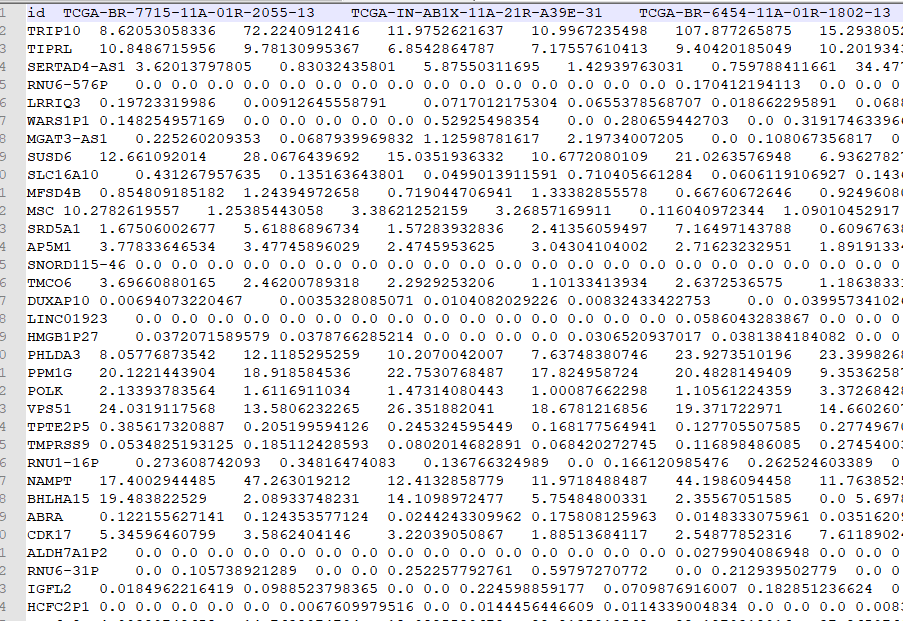
rt=as.matrix(rt)
rownames(rt)=rt[,1]
exp=rt[,2:ncol(rt)]
# 将处理后的矩阵中的表达数据提取出来。
dimnames=list(rownames(exp),colnames(exp))
#创建矩阵 data 设置维度名称。
#在R中,维度名称允许你为矩阵的行和列指定标签,以便更好地理解矩阵中的数据
data=matrix(as.numeric(as.matrix(exp)),nrow=nrow(exp),dimnames=dimnames)
#将数据矩阵 exp 转换为 data 矩阵。具体步骤如下:
# as.matrix(exp): 将数据框 exp 转换为数值型矩阵。
# as.numeric(...): 将数值型矩阵中的数据转换为数值。
# matrix(...): 使用转换后的数值和指定的行数(由 nrow(exp) 给出)和维度名称(由 dimnames 给出)创建一个新的矩阵 data。
#删掉正常样品
data=avereps(data)
#函数 avereps 对矩阵 data 进行处理。avereps 函数的目的是求每一行的平均值
data=data[rowMeans(data)>0.5,]
#将矩阵 data 进一步处理,只保留行平均值大于0.5的行。
#排除那些平均表达量较低的样本,以便更关注表达丰度较高的样本。
group=sapply(strsplit(colnames(data),"\-"), "[", 4)
#将矩阵 data 的列名进行处理,提取出每列名称的第四个部分(使用 "-" 作为分隔符)。用于从样本名中获取样本的分组信息。
group=sapply(strsplit(group,""), "[", 1)
#将每个字符串中的每个字符作为单独的元素。然后,使用 [ 函数提取每个字符串的第一个字符。这可能是为了进一步处理样本分组信息。
group=gsub("2","1",group)
#用 gsub 函数将所有字符串中的字符 "2" 替换为 "1"。这可能是将样本分组信息进行标准化或重新编码。
data=data[,group==0]
#将矩阵 data 进一步处理,只保留样本分组信息为 "0" 的列。这可能是为了只保留特定样本分组的数据。
data=t(data)
#对矩阵 data 进行转置,将行列互换
rownames(data)=gsub("(.*?)\-(.*?)\-(.*?)\-.*", "\1\-\2\-\3", rownames(data))
#用正则表达式处理矩阵 data 的行名,从中提取出所需的信息,并重新设置行名。
data=t(avereps(data))
#根据目标基因的表达量对样品进行分组
geneExp=as.data.frame(t(data[gene,,drop=F]))
geneExp$Type=ifelse(geneExp[,gene]>median(geneExp[,gene]), "High", "Low")
#从矩阵 data 中选择与目标基因相关的行,
#并使用逗号 , 来指定列的选择。drop=F 参数确保即使只选择一列,结果也会保持为矩阵而不是向量。
for(drug in allDrugs){
#预测药物敏感性
possibleError=tryCatch(
{senstivity=pRRopheticPredict(data, drug, selection=1, dataset = "cgp2016")},
error=function(e) e)
#使用 tryCatch 函数,尝试执行药物敏感性预测。
#如果在执行过程中出现错误,将在错误处理函数中捕获错误并存储在 possibleError 中。
if(inherits(possibleError, "error")){next}
#如果 possibleError 包含了一个错误,那么这行代码将跳过当前药物,继续下一个循环迭代。
senstivity=senstivity[senstivity!="NaN"]
#移除药物敏感性结果中的 "NaN" 值,将有效的药物敏感性值存储在变量 senstivity 中。
senstivity[senstivity>quantile(senstivity,0.99)]=quantile(senstivity,0.99)
#对药物敏感性值进行处理,将高于 0.99 分位数的值设置为 0.99。这可能是为了去除极端异常值,以获得更好的可视化效果
#将表达数据与药物敏感性的结果进行合并
sameSample=intersect(row.names(geneExp), names(senstivity))
#找到 geneExp 中与药物敏感性结果具有相同样本名称的样本。
geneExp=geneExp[sameSample, "Type",drop=F]
#从 geneExp 中选择与药物敏感性结果相同的样本,仅保留 "Type" 列。
senstivity=senstivity[sameSample]
#从药物敏感性结果中选择与 geneExp 相同样本的敏感性值。
rt=cbind(geneExp, senstivity)
#将基因表达分组和药物敏感性结果合并到一个数据框中。
#设置比较组
rt$Type=factor(rt$Type, levels=c("Low", "High"))
#将 "Type" 列转换为因子,设定水平为 "Low" 和 "High"。
type=levels(factor(rt[,"Type"]))
comp=combn(type, 2)
my_comparisons=list()
#创建一个空列表来存储分组比较
for(i in 1:ncol(comp)){my_comparisons[[i]]<-comp[,i]}
#将所有可能的分组比较添加到 my_comparisons 列表中。
#获取高低表达组差异的pvalue
test=wilcox.test(senstivity~Type, data=rt)
#执行 Wilcoxon 秩和检验,检验药物敏感性是否在不同基因表达分组之间存在显著差异。
diffPvalue=test$p.value
if(diffPvalue <pFilter){< span>
</pFilter){<>
#绘制箱线图
boxplot=ggboxplot(rt, x="Type", y="senstivity", fill="Type",
xlab=gene,
ylab=paste0(drug, " senstivity (IC50)"),
legend.title=gene,
palette=c("#0066FF","#FF0000")
)+
stat_compare_means(comparisons=my_comparisons)
pdf(file=paste0("durgSenstivity.", drug, ".pdf"), width=5, height=4.5)
#创建一个 PDF 文件,用于保存绘制的箱线图,文件名根据当前药物命名。
print(boxplot)
dev.off()
}
}
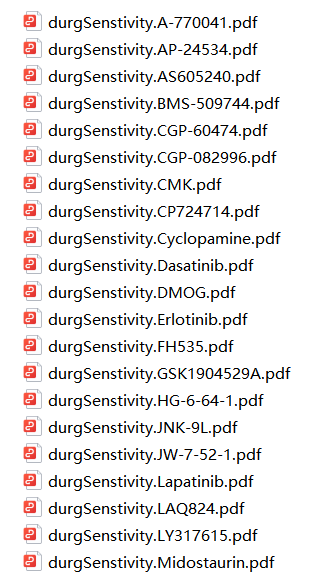
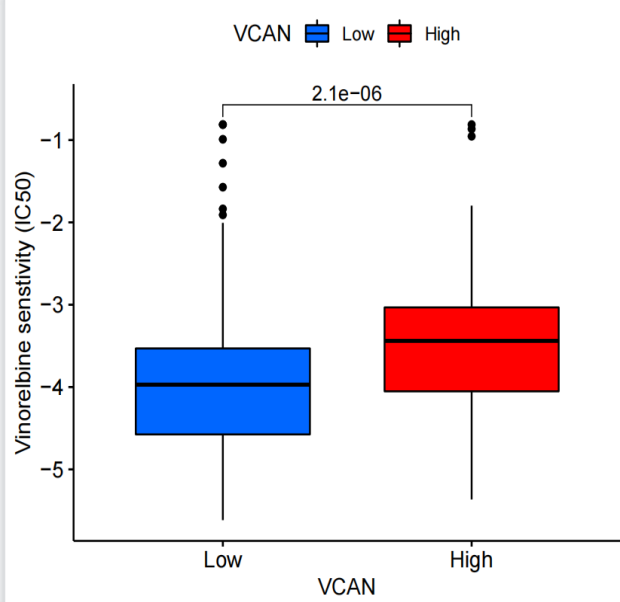
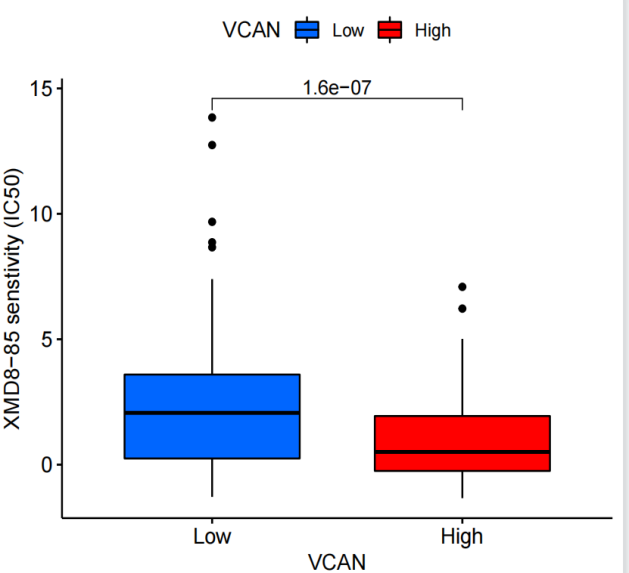
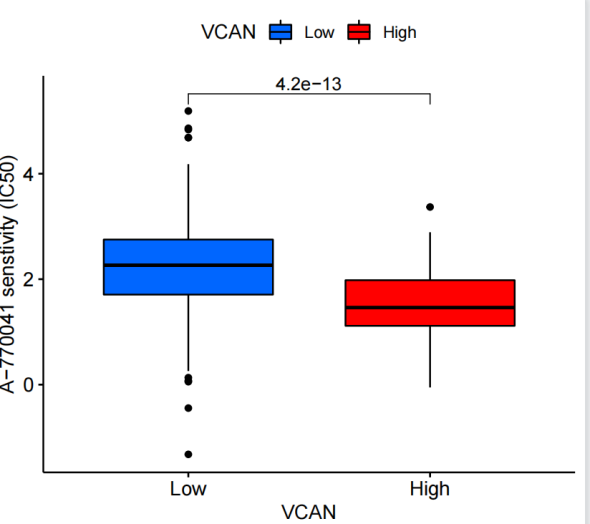
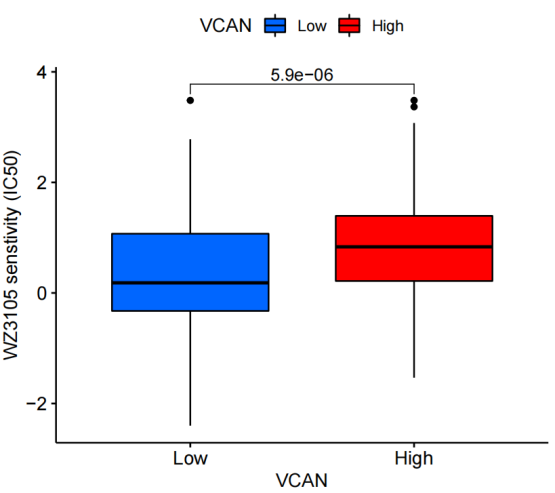
library(reshape2)
library(ggpubr)
th=theme(axis.text.x = element_text(angle = 45,vjust = 0.5))
dir='./DataFiles/Training Data/'
GDSC2_Expr = readRDS(file=file.path(dir,'GDSC2_Expr (RMA Normalized and Log Transformed).rds'))
dim(GDSC2_Expr)
GDSC2_Expr[1:4, 1:4]
boxplot(GDSC2_Expr[,1:4])
df=melt(GDSC2_Expr[,1:4])
head(df)
p1=ggboxplot(df, "Var2", "value") +th
# Read GDSC2 response data. rownames() are samples, colnames() are drugs.
dir
GDSC2_Res = readRDS(file = file.path(dir,"GDSC2_Res.rds"))
dim(GDSC2_Res) # 805 198
GDSC2_Res[1:4, 1:4]
p2=ggboxplot(melt(GDSC2_Res[ , 1:4]), "Var2", "value") +th ; p2# IMPORTANT note: here I do e^IC50 since the IC50s are actual ln values/log transformed already, and the calcPhenotype function Paul #has will do a power transformation (I assumed it would be better to not have both transformations)
GDSC2_Res <- exp(GDSC2_Res)
p3=ggboxplot(melt(GDSC2_Res[ , 1:4]), "Var2", "value") +th ; p3
library(patchwork)
p1+p2+p3
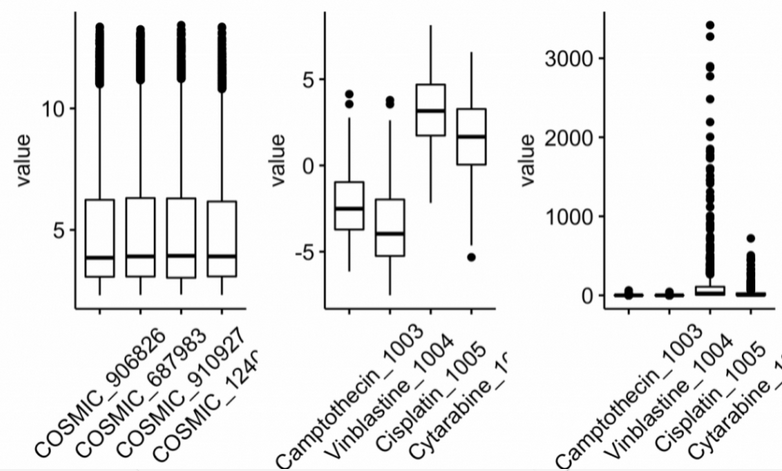
ggboxplot(melt(GDSC2_Res[ 1:4 ,]), "Var1", "value") +th
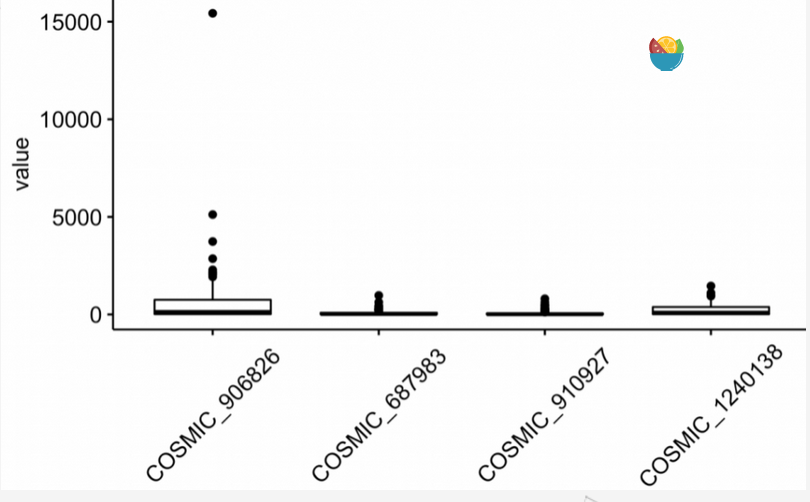
round(apply(GDSC2_Res[ 1:4 ,], 1, function(x){
return(c(
head(sort(x)),
tail(sort(x))
))
}),2)
每个细胞系都是有自己的特异性药物和废物药物,IC50接近于0的就是神药 。表达量矩阵是从Genomics of Drug Sensitivity in Cancer (GDSC) 的
v2里面随机挑选10个细胞系作为要预测的矩阵。读入训练集的表达量矩阵 和药物处理信息。
install.packages("oncoPredict")
rm(list = ls()) ## 魔幻操作,一键清空~
options(stringsAsFactors = F)
library(oncoPredict)
library(data.table)
library(gtools)
library(reshape2)
library(ggpubr)
th=theme(axis.text.x = element_text(angle = 45,vjust = 0.5))
dir='./DataFiles/Training Data/'
GDSC2_Expr = readRDS(file=file.path(dir,'GDSC2_Expr (RMA Normalized and Log Transformed).rds'))
GDSC2_Res = readRDS(file = file.path(dir,"GDSC2_Res.rds"))
GDSC2_Res <- exp(GDSC2_Res)
testExpr<- GDSC2_Expr[,sample(1:ncol(GDSC2_Expr),10)]testExpr[1:4,1:4]
colnames(testExpr)=paste0('test',colnames(testExpr))
dim(testExpr)
calcPhenotype(trainingExprData = GDSC2_Expr,
trainingPtype = GDSC2_Res,
testExprData = testExpr,
batchCorrect = 'eb', # "eb" for ComBat
powerTransformPhenotype = TRUE,
removeLowVaryingGenes = 0.2,
minNumSamples = 10,
printOutput = TRUE,
removeLowVaringGenesFrom = 'rawData' )
#解读药物预测结果
library(data.table)testPtype <- fread('./calcPhenotype_Output/DrugPredictions.csv', data.table = F)testPtype[1:4, 1:4]
小果还提供思路设计、定制生信分析、文献思路复现;有需要的小伙伴欢迎直接扫码咨询小果,竭诚为您的科研助力!
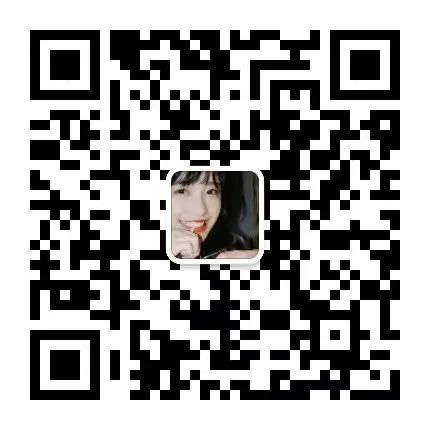
定制生信分析
服务器租赁
扫码咨询小果
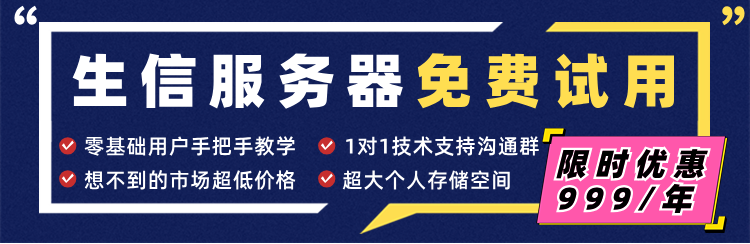
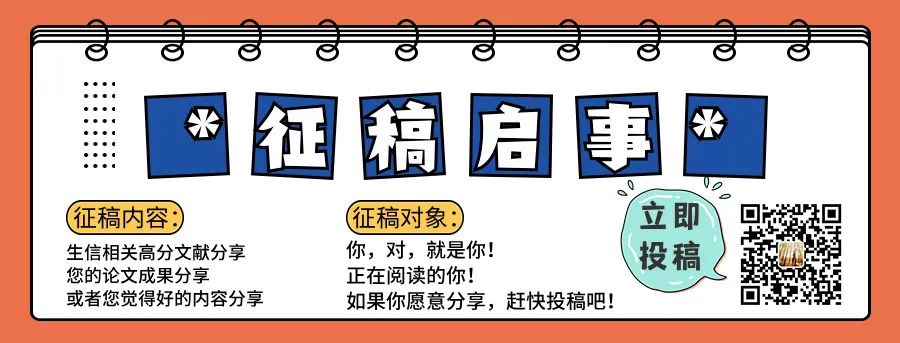
往期回顾
01 |
02 |
03 |
04 |