机器学习难入门?和小果一起来学学tidymodels包
在生信研究的过程中,我们可以分为两大方向,一个是对数据进行统计分析,一个是为统计分析创建工具,也就是模型算法的研发和构建。打个比方:在疾病预测的研究中,我们可能回想讨论某个因素与致死率相关性有多少,是一个回归问题,那么我们就会用到模型进行拟合;在转录组的分析中,聚类是一个重点,聚类则为一个分类问题,需要用到分类模型。在大多数小伙伴的学习中,我们都是工具的使用者,不知道有没有对创造工具有兴趣的小伙伴呀?今天小果想为大家介绍一个整合性质的R包,选择了tidyverse的思路,这个R包就是tidymodels!今天小果想带着大家先有一个简单的认识,和大家一起来建立一个模型吧。
step0 安装与载入R包
if(!require(tidymodels)) install.packages("tidymodels")
if(!require(broom.mixed)) install.packages("broom.mixed")
if(!require(dotwhisker)) install.packages("dotwhisker")
library(tidymodels)
library(readr) # 输入数据
library(broom.mixed) # 将贝叶斯模型转换为tibbles类型
library(dotwhisker) # 用于可视化回归结果
step1 数据准备
首先,让我们将海胆数据读入 R,我们将通过提供readr::read_csv()CSV 数据所在的 url 来完成此操作(“ https://tidymodels.org/start/models/urchins.csv ”)。在进行建模时,对数据有前沿认识也是十分重要的哦!这组海胆的数据探索了三种不同的喂养方式如何随着时间的推移影响海胆的大小。实验开始时海胆的初始大小可能会影响它们在进食时的生长大小。food_regime:实验喂养方案 initial_volume:初始大小 width:宽度
urchins <-
read_csv("https://tidymodels.org/start/models/urchins.csv") %>%
setNames(c("food_regime", "initial_volume", "width")) %>%
mutate(food_regime =factor(food_regime, levels =c("Initial", "Low", "High")))
urchins
# # A tibble: 72 × 3
# food_regime initial_volume width
#
# 1 Initial 3.5 0.01
# 2 Initial 5 0.02
# 3 Initial 8 0.061
# 4 Initial 10 0.051
# 5 Initial 13 0.041
# 6 Initial 13 0.061
# 7 Initial 15 0.041
# 8 Initial 15 0.071
# 9 Initial 16 0.092
# 10 Initial 17 0.051
# # ℹ 62 more rows
我们对数据进行一下可视化,了解一下数据的具体分布。我们可以看到,实验喂养方式的改变以及初始的体积均会影响海胆的宽度。
initial_volume, =
y = width,
group = food_regime,
col = food_regime)) +
+
lm, se =FALSE) + =
"plasma", end = .7) =
需要租赁服务器的小伙伴可以扫码添加小果,此外小果还提供生信分析,思路设计,文献复现等,有需要的小伙伴欢迎来撩~
step2 构建模型
那么我们对于这个数据,在回归与分类两个中进行选择,我们应该选择线性回归模型进行拟合分析。
在这小果选择了parsnip包的线性回归模型
linear_reg() # 默认模型使用的是最小二乘法
# Linear Regression Model Specification (regression)
#
# Computational engine: lm
保存模型
lm_mod <-linear_reg()
使用fit函数估计或训练模型
lm_fit <-
lm_mod %>%
fit(width ~ initial_volume * food_regime, data = urchins)
lm_fit
# parsnip model object
#
#
# Call:
# stats::lm(formula = width ~ initial_volume * food_regime, data = data)
#
# Coefficients:
# (Intercept) initial_volume
# 0.0331216 0.0015546
# food_regimeLow food_regimeHigh
# 0.0197824 0.0214111
# initial_volume:food_regimeLow initial_volume:food_regimeHigh
# -0.0012594 0.0005254
许多模型都有 tidy函数,区别于summary函数,以一种更可预测和更有用的格式提供摘要结果哦!(例如具有标准列名称的数据框)
tidy(lm_fit)
# # A tibble: 6 × 5
# term estimate std.error statistic p.value
#
# 1 (Intercept) 0.0331 0.00962 3.44 0.00100
# 2 initial_volume 0.00155 0.000398 3.91 0.000222
# 3 food_regimeLow 0.0198 0.0130 1.52 0.133
# 4 food_regimeHigh 0.0214 0.0145 1.47 0.145
# 5 initial_volume:food_regimeLow -0.00126 0.000510 -2.47 0.0162
# 6 initial_volume:food_regimeHigh 0.000525 0.000702 0.748 0.457
在得出结果后,小果使用了dotwhisker对回归的结果生成点须图。点为estimate的值,线为误差线。
tidy(lm_fit) %>%
dwplot(dot_args =list(size =2, color ="black"),
whisker_args =list(color ="black"),
vline =geom_vline(xintercept =0, colour ="grey50", linetype =2))
step3 构建测试数据集
new_points <-expand.grid(initial_volume =20,
food_regime =c("Initial", "Low", "High"))
new_points
# initial_volume food_regime
# 1 20 Initial
# 2 20 Low
# 3 20 High
step4 预测数据
mean_pred <-predict(lm_fit, new_data = new_points)
mean_pred
# # A tibble: 3 × 1
# .pred
#
# 1 0.0642
# 2 0.0588
# 3 0.0961
添加置信空间
conf_int_pred <-predict(lm_fit,
new_data = new_points,
type ="conf_int")
conf_int_pred
# # A tibble: 3 × 2
# .pred_lower .pred_upper
#
# 1 0.0555 0.0729
# 2 0.0499 0.0678
# 3 0.0870 0.105
准备绘图数据
plot_data <-
new_points %>%
bind_cols(mean_pred) %>%
bind_cols(conf_int_pred)
将置信空间的预测值作为误差线
ggplot(plot_data, aes(x = food_regime)) +
geom_point(aes(y = .pred)) +
geom_errorbar(aes(ymin = .pred_lower,
ymax = .pred_upper),
width = .2) +
labs(y ="urchin size")
由于模型的构建对大多数0基础的小伙伴来说可能有点难接受,但没有关系哦,跟着小果先把代码运行出来,我们后期再一步一步的分析代码,到底为什么这么做呢?还可以怎么细调参数进行改正呢?今天小果和大家分享的只是tidymodels的一小部分,这个R包还有很多别的功能,我们可以用它进行数据划分,数据的预处理等等一系列机器学习的函数。有兴趣的小伙伴可以关注一下小果,小果会继续更新哦~那么今天小果的分享就到这里啦!
小果还提供思路设计、定制生信分析、文献思路复现;有需要的小伙伴欢迎直接扫码咨询小果,竭诚为您的科研助力!
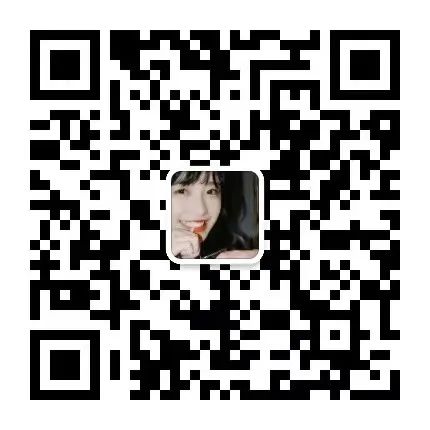
定制生信分析
服务器租赁
扫码咨询小果
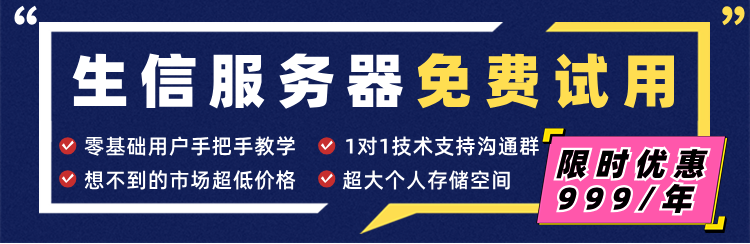
往期回顾
01 |
02 |
03 |
04 |